“Generative AI” has arguably been the most used buzzword in tech and mainstream media. It basically means that the AI can “generate” content based on existing data sets.
The technology is already found in different niches. Marketers use it to automate copywriting, creatives use it to generate designs, and programmers are writing code faster than ever.
But what exactly is generative AI, how can you use it in your own business, and what are the limitations you should look out for? Let’s get right into it.
What is Generative AI?
Generative AI, or Gen AI, enables users to quickly generate new content based on inputs, including text, images, music, videos, or other types of data. The input, also called a prompt, is simply an instruction you give to the AI algorithm to receive an output.
Generative AI, in a way, learns the way we do. We take inspiration from art, learn things at school, and apply the knowledge in our bodies of work. It takes data and gives creativity.
Artificial intelligence is nothing new; it’s been around for a while. So what’s with all the buzz? What we’ve known in the past was traditional AI, a rule-based system that focused on tasks like classification and prediction. Think of recommendation systems that use machine learning to give users personalized product recommendations or spam filters that analyze emails and flag anything that looks fishy.
And then you have generative AI—the creative maverick. Gen AI is not limited to structured data and predefined algorithms. Instead of just following rules, it uses patterns and data to generate content—be it text, images, or even music. It’s all about making something fresh from the existing stuff.
So, in a nutshell, the difference between the two lies in the following: Traditional AI could help you find the best hotel prices or the quickest route to the next McDonald’s. Generative AI can compose music, paint pictures, and even craft prose.
Key Features of Generative AI
Generative AI can learn fast and give you output in seconds. But, the key features of all these tools are anchored on the following:
Creativity and Innovation
Humans are great at using tools. We used it for art, science, and every medium you can think of. Generative AI is like that—tools we can use to elevate our creativity and innovation.
Let’s say you have a character idea for a fantasy story but not that good at drawing. You can create a rough sketch, and generative AI can create a character based on your idea.
Adaptability in Various Contexts
AI is incredibly good at iteration. If you want generative AI to learn, all you have to do is present it with the right context and the right data. This is possible thanks to advancements in LLMs.
Large Language Models help AI contextualize what we want it to do. You can even assign roles to AI. For example, you can tell the AI that it’s a customer support agent for your business. It will then align its response to fit that role and contextualize input from users.
Feedback Loops
Generative AI improves over time by learning from its outputs. If something doesn’t hit the mark, it adjusts based on feedback. That means that the output you receive is not set in stone. If you don’t like it, or it’s not quite what you were looking for, provide feedback and additional context that will help Gen AI give you the answer you need.
The best part? Gen AI will use this feedback to improve its output, and with every iteration, you can expect better results.
Interactivity
Generative AI thrives when users can input prompts or parameters, making it feel like a two-way street. This is where it gets fun—whether you’re asking it to write a poem or create an image, the back-and-forth can lead to some wild and unexpected results. Interactivity is the key to output that contains your own flair.
Fundamentals of Generative AI
To understand how generative AI works, we need to understand its learning mechanisms. When you know how AI learns, you can optimize how it’s used for your specific needs.
How Generative AI Learns
Most AI learn using machine learning or deep learning. Machine learning looks at patterns in data sets and predicts outputs based on what it learns. But here’s the kicker: generative AI takes this to the next level by digging deep into vast amounts of data, identifying intricate patterns, and generating outputs that aren’t just repeats but fresh creations. This ability to learn from data and make new content is what fuels the magic of generative AI.
AI uses deep learning and artificial neural networks to mimic how the human brain processes data. Imagine a web of interconnected neurons firing off signals—this is essentially how neural networks work. By layering these networks, generative AI can build a complex understanding of context. This makes the output contextually relevant and coherent. So when you prompt it with a question or a task, it’s not just spitting out random words, it’s crafting responses that make sense in the given context. That’s why Gen AI is the perfect partner to riff off your ideas.
Types of Data Sets Required
Generative AI needs to learn from high-quality, diverse datasets. You’d typically need training, validation, and testing data sets. While there’s no strict rule, the rough standard for train-validation-test splits is 60-80% training data, 10-20% validation data, and 10-20% test data.
Training data sets are used to train the generative AI model and teaches the algorithm what to look for in the data. The quality and diversity of this data matter a ton. Feed it junk, and you can expect junk in return. It’s a classic case of “you are what you eat,” so if you want gourmet results, you better start with top-notch ingredients.
Validation data sets are made up of known data. It’s used to validate and evaluate the parameters of the model during training. The training phase is when the real magic happens. This is when algorithms and generative models, like GANs and VAEs, go to work, analyzing the data to learn patterns and styles (more on them later).
The test data set is the output. It shows how much your model has learned from the previous datasets. After the initial training and feedback, the AI undergoes fine-tuning to specialize in specific tasks or styles, whether that’s crafting witty blog posts or generating stunning artwork.
Real-World Applications of Generative AI
Generative AI is already making waves in various fields. Many industries are on the cusp of major change—for the better. According to McKinsey, respondents most often report generative AI adoption in their marketing and sales, product and service development, and IT functions.
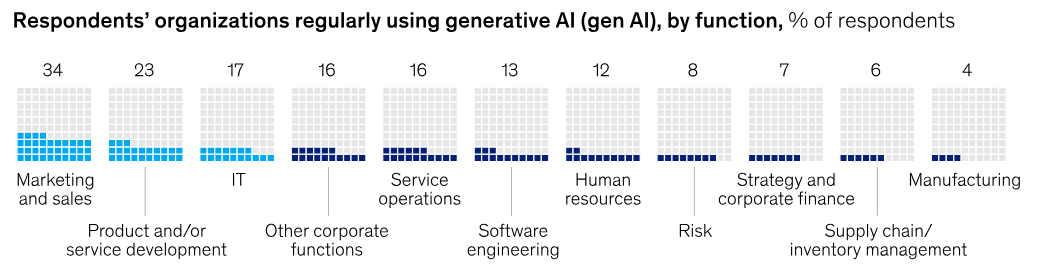
This is proof that generative AI is not a passing fad and that it will irrevocably shake things up in various industries. Here are some standout examples:
AI in Creative Arts
Generative AI is already finding its place in creative arts, music, and literature. Tools like OpenAI's DALL-E can generate images from textual descriptions, bridging the gap between written and visual creativity. Generative AI has become a game-changer for artists, designers, and anyone with a flair for creativity. What was unimaginable in the past is now possible with tools like DALL-E that can take a simple text prompt and turn it into stunning visuals.
Text Generation Tools
LLMs are probably the most known types of AI generative AI. These tools can generate emails, product descriptions, and entire articles. Marketers can use these tools to streamline and automate workflows by reducing manual data tasks. This doesn’t just save time—it frees up creative minds to focus on the big ideas instead of getting bogged down in the nitty-gritty.
Healthcare
In healthcare, generative AI is aiding in drug discovery and medical imaging. The best example of this is Generative Adversarial Networks (GANs). Researchers use GANs to create synthetic medical images, enhance data quality, and improve disease detection. Thanks to gen AI, researchers are generating synthetic medical data for training algorithms, which is essential when real patient data is scarce or sensitive.
Finance
Massive financial institutions are using generative AI to detect fraud. Generative AI can analyze transaction data to generate simulations of normal and fraudulent behavior. By learning from patterns of past fraudulent activities, it can flag suspicious transactions before they even happen. Moreover, with the use of AI, financial institutions can make market trend predictions and algorithmic trading. Smaller businesses can use generative AI for sales forecasting, risk analysis, and portfolio optimizations.
Entertainment
AI is also becoming a standard in entertainment. For example, generative AI in the video game industry can be used to create contextual dynamic dialogue between a user and an NPC or be used to create design elements within the game. Talking about video game development, think about how frustrating it can be to run into repetitive landscapes in a game. With generative AI, developers can create vast, diverse worlds that feel alive and ever-changing.
Customer service
Chatbots powered by generative AI are taking customer interactions to the next level. Gone are the days of rigid, scripted responses that make you want to pull your hair out. Now, we’ve got chatbots that can engage in natural, human-like conversations. They understand context, respond appropriately, and even pick up on customer sentiment. Then there’s the capability for 24/7 support. Generative AI doesn’t sleep or take coffee breaks, meaning customers can get help whenever they need it.
Techniques in Generative AI
There are different types of artificial intelligence, such as generative AI, predictive AI, and conversational AI. The difference lies in the techniques they use and the output they provide. In this section, we’ll dive deeper into the techniques used for generative AI.
Generative Adversarial Networks (GANs)
Generative Adversarial Networks (GANs) are a groundbreaking framework in the world of generative AI, designed to create new data by harnessing the power of two competing neural networks in a battle of wits: the generator and the discriminator.
The generator's job is to produce synthetic data—think images, music, or text—while the discriminator acts as a critic, evaluating the authenticity of the generated data against real data from the training set. This adversarial process is akin to a game where the generator strives to outsmart the discriminator, continually improving its outputs to create increasingly realistic content.
Over time, this dynamic pushes the generator to produce data that’s often indistinguishable from genuine examples, making GANs a powerful tool for applications ranging from deepfake creation to enhancing image resolution and beyond.
Variational Autoencoders (VAEs)
Variational Autoencoders (VAEs) are designed to take complex data and make it more manageable. Simply put, a VAE is a smarter autoencoder. It learns to organize its data in a way that makes it easier to create new, realistic-looking data points.
Think of a VAE as a mechanism that can compress intricate information into a simpler form—essentially creating a lower-dimensional representation of the data—while still preserving its essential characteristics. The "variational" part comes from a connection to a statistical method, but don't worry about that too much. The key takeaway is that VAEs are great for generating high-quality data that looks like the real thing.
Transformer Models
Transformer models like GPT-3 and GPT-4 got AI into the mainstream. They use natural language processing (NLP) to create text that’s so good, you'd swear it was written by a human.
These models are built on a special architecture called the transformer, which helps them understand nuance, like how different words in a sentence relate to each other. This gives them the power to create text that's not only grammatically correct but also makes sense in context. This technique powers everything from chatbots to content generation, making it a cornerstone of modern generative AI applications.
Reinforcement Learning
Reinforcement learning is all about learning from the pros—us humans! In reinforcement learning, generative models improve based on feedback provided by people, allowing them to align their outputs with human values and preferences. It’s like having an editor read your drafts and tell you what works and what totally doesn’t. This method ensures that the AI doesn’t just spit out random content but creates responses that resonate with real human needs, making interactions more meaningful and less robotic.
Think of Netflix’s recommendation system. After watching a show, you can either Like it or Dislike it. Netflix takes that into account, shows you less of what you don’t want to watch, and shows more of the show you like.
Future Trends and Impact
Generative AI is scary good. It’s not just about text anymore. With better multimodal capabilities in newer models, AI can work with voice, image, and videos.
Data from McKinsey shows that the adoption of AI among organizations in at least one business function has jumped to 72%. While the adoption rate of generative AI among organizations sits at 65%, we’ve witnessed a radical jump from just 33% in 2023.

If we zoom in on the profile of generative AI users, we can see that 65% are Millennials or Gen Z, and 72% are employed. When it comes to the use cases, 75% of generative AI users are looking to automate tasks at work and use generative AI for work communications.
Even though these are staggering statistics, there’s no doubt that we’ve only scratched the surface of generative AI. Gen AI tools are already cranking out all kinds of content—from written pieces and stunning images to videos, audio, and even code. In the near future we can expect applications that target specific industries and functions, that will completely revolutionize productivity.
According to McKinsey, gen AI will perform at a median level of human performance by the end of this decade. And its performance will compete with the top 25% of people completing any task before 2040.
Models like Meta’s Llama 3.1 already boast 128K context length. That means AI is more capable of understanding context, giving faster responses, and being more accurate with output.
Generative AI: The Next Frontier
Generative AI is getting better at an unprecedented rate. If you’re looking for the best generative AI tool that’s built for copywriting, Jasper.ai is a must-try. Start automating your content marketing today for free with Jasper.